Hyperexponential distribution Contents Properties Fitting See also References Navigation...
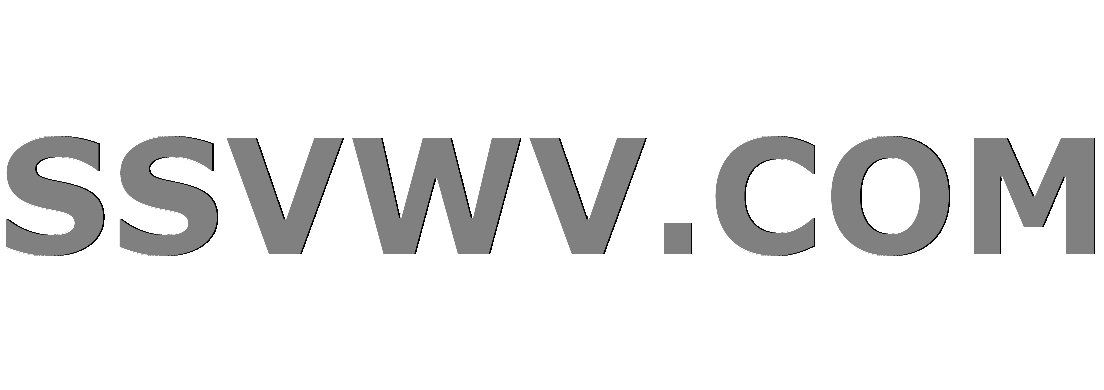
Multi tool use
BenfordBernoullibeta-binomialbinomialcategoricalhypergeometricPoisson binomialRademachersolitondiscrete uniformZipfZipf–MandelbrotarcsineARGUSBalding–NicholsBatesbetabeta rectangularIrwin–HallKumaraswamylogit-normalnoncentral betaraised cosinereciprocaltriangularU-quadraticuniformWigner semicircleCauchyexponential powerFisher's zGaussian qgeneralized normalgeneralized hyperbolicgeometric stableGumbelHoltsmarkhyperbolic secantJohnson's SULandauLaplaceasymmetric Laplacelogisticnoncentral tnormal (Gaussian)normal-inverse Gaussianskew normalslashstableStudent's ttype-1 GumbelTracy–Widomvariance-gammaVoigtrectified GaussianCircularcompound Poissonellipticalexponentialnatural exponentiallocation–scalemaximum entropymixturePearsonTweediewrapped
Continuous distributions
probability theorycontinuous probability distributionprobability density functionrandom variableexponentially distributedcoefficient of variationhypoexponential distributionexponential distributiongeometric distributionhypergeometric distributionmixture densitytelephonycoefficient of variationmoment-generating functionheavy-tailed distributionProny's method

Diagram showing queueing system equivalent of a hyperexponential distribution
In probability theory, a hyperexponential distribution is a continuous probability distribution whose probability density function of the random variable X is given by
- fX(x)=∑i=1nfYi(x)pi,{displaystyle f_{X}(x)=sum _{i=1}^{n}f_{Y_{i}}(x);p_{i},}
where each Yi is an exponentially distributed random variable with rate parameter λi, and pi is the probability that X will take on the form of the exponential distribution with rate λi.[1] It is named the hyperexponential distribution since its coefficient of variation is greater than that of the exponential distribution, whose coefficient of variation is 1, and the hypoexponential distribution, which has a coefficient of variation smaller than one. While the exponential distribution is the continuous analogue of the geometric distribution, the hyperexponential distribution is not analogous to the hypergeometric distribution. The hyperexponential distribution is an example of a mixture density.
An example of a hyperexponential random variable can be seen in the context of telephony, where, if someone has a modem and a phone, their phone line usage could be modeled as a hyperexponential distribution where there is probability p of them talking on the phone with rate λ1 and probability q of them using their internet connection with rate λ2.
Contents
1 Properties
2 Fitting
3 See also
4 References
Properties
Since the expected value of a sum is the sum of the expected values, the expected value of a hyperexponential random variable can be shown as
- E[X]=∫−∞∞xf(x)dx=∑i=1npi∫0∞xλie−λixdx=∑i=1npiλi{displaystyle E[X]=int _{-infty }^{infty }xf(x),dx=sum _{i=1}^{n}p_{i}int _{0}^{infty }xlambda _{i}e^{-lambda _{i}x},dx=sum _{i=1}^{n}{frac {p_{i}}{lambda _{i}}}}
and
- E[X2]=∫−∞∞x2f(x)dx=∑i=1npi∫0∞x2λie−λixdx=∑i=1n2λi2pi,{displaystyle E!left[X^{2}right]=int _{-infty }^{infty }x^{2}f(x),dx=sum _{i=1}^{n}p_{i}int _{0}^{infty }x^{2}lambda _{i}e^{-lambda _{i}x},dx=sum _{i=1}^{n}{frac {2}{lambda _{i}^{2}}}p_{i},}
from which we can derive the variance:[2]
- Var[X]=E[X2]−E[X]2=∑i=1n2λi2pi−[∑i=1npiλi]2=[∑i=1npiλi]2+∑i=1n∑j=1npipj(1λi−1λj)2.{displaystyle operatorname {Var} [X]=E!left[X^{2}right]-E!left[Xright]^{2}=sum _{i=1}^{n}{frac {2}{lambda _{i}^{2}}}p_{i}-left[sum _{i=1}^{n}{frac {p_{i}}{lambda _{i}}}right]^{2}=left[sum _{i=1}^{n}{frac {p_{i}}{lambda _{i}}}right]^{2}+sum _{i=1}^{n}sum _{j=1}^{n}p_{i}p_{j}left({frac {1}{lambda _{i}}}-{frac {1}{lambda _{j}}}right)^{2}.}
The standard deviation exceeds the mean in general (except for the degenerate case of all the λs being equal), so the coefficient of variation is greater than 1.
The moment-generating function is given by
- E[etx]=∫−∞∞etxf(x)dx=∑i=1npi∫0∞etxλie−λixdx=∑i=1nλiλi−tpi.{displaystyle E!left[e^{tx}right]=int _{-infty }^{infty }e^{tx}f(x),dx=sum _{i=1}^{n}p_{i}int _{0}^{infty }e^{tx}lambda _{i}e^{-lambda _{i}x},dx=sum _{i=1}^{n}{frac {lambda _{i}}{lambda _{i}-t}}p_{i}.}
Fitting
A given probability distribution, including a heavy-tailed distribution, can be approximated by a hyperexponential distribution by fitting recursively to different time scales using Prony's method.[3]
See also
- Phase-type distribution
- Hyper-Erlang distribution
Lomax distribution (continuous mixture of exponentials)
References
^ Singh, L. N.; Dattatreya, G. R. (2007). "Estimation of the Hyperexponential Density with Applications in Sensor Networks". International Journal of Distributed Sensor Networks. 3 (3): 311. doi:10.1080/15501320701259925..mw-parser-output cite.citation{font-style:inherit}.mw-parser-output .citation q{quotes:"""""""'""'"}.mw-parser-output .citation .cs1-lock-free a{background:url("//upload.wikimedia.org/wikipedia/commons/thumb/6/65/Lock-green.svg/9px-Lock-green.svg.png")no-repeat;background-position:right .1em center}.mw-parser-output .citation .cs1-lock-limited a,.mw-parser-output .citation .cs1-lock-registration a{background:url("//upload.wikimedia.org/wikipedia/commons/thumb/d/d6/Lock-gray-alt-2.svg/9px-Lock-gray-alt-2.svg.png")no-repeat;background-position:right .1em center}.mw-parser-output .citation .cs1-lock-subscription a{background:url("//upload.wikimedia.org/wikipedia/commons/thumb/a/aa/Lock-red-alt-2.svg/9px-Lock-red-alt-2.svg.png")no-repeat;background-position:right .1em center}.mw-parser-output .cs1-subscription,.mw-parser-output .cs1-registration{color:#555}.mw-parser-output .cs1-subscription span,.mw-parser-output .cs1-registration span{border-bottom:1px dotted;cursor:help}.mw-parser-output .cs1-ws-icon a{background:url("//upload.wikimedia.org/wikipedia/commons/thumb/4/4c/Wikisource-logo.svg/12px-Wikisource-logo.svg.png")no-repeat;background-position:right .1em center}.mw-parser-output code.cs1-code{color:inherit;background:inherit;border:inherit;padding:inherit}.mw-parser-output .cs1-hidden-error{display:none;font-size:100%}.mw-parser-output .cs1-visible-error{font-size:100%}.mw-parser-output .cs1-maint{display:none;color:#33aa33;margin-left:0.3em}.mw-parser-output .cs1-subscription,.mw-parser-output .cs1-registration,.mw-parser-output .cs1-format{font-size:95%}.mw-parser-output .cs1-kern-left,.mw-parser-output .cs1-kern-wl-left{padding-left:0.2em}.mw-parser-output .cs1-kern-right,.mw-parser-output .cs1-kern-wl-right{padding-right:0.2em}
^ H.T. Papadopolous; C. Heavey; J. Browne (1993). Queueing Theory in Manufacturing Systems Analysis and Design. Springer. p. 35. ISBN 9780412387203.
^ Feldmann, A.; Whitt, W. (1998). "Fitting mixtures of exponentials to long-tail distributions to analyze network performance models" (PDF). Performance Evaluation. 31 (3–4): 245. doi:10.1016/S0166-5316(97)00003-5.
hKQVFhrQa2 56rWGsPQ85qd K28X GgvrYBvkDHehsNtQgryrdssZqcoKIO,h 00 Uz,Kgwlklj